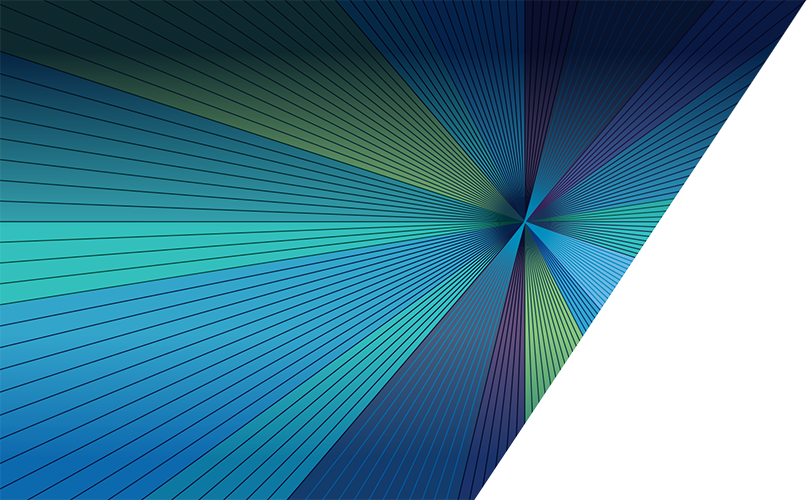
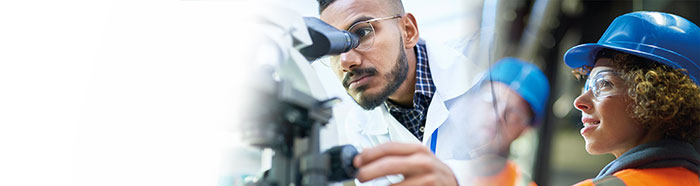
Research projects
- Research area
Big data sensors and digitalisation for the offshore environment
- Institution
Durham University
- Research project
Data-driven Infrastructure Planning for Offshore Wind Farms
- Lead supervisor
Dr Behzad Kazemtabrizi (Associate Professor in Electrical Engineering, Durham University)
- PhD Student
- Supervisory Team
Professor Christopher Crabtree (Professor – Wind Energy Systems, Durham University)
Professor Matthias Troffaes (Professor, Probability - Department of Mathematical Sciences, Durham University, Durham University)
Project Description:
The UK’s share of offshore wind energy has been steadily increasing in recent years; there is now 10.4 GW of installed capacity offshore (reference: UK Wind Energy Database online). There are already plans for developing large-scale offshore wind farms with capacities exceeding 1 GW. There remains however a degree of uncertainty over how to best develop, maintain and operate the wind farms and their underlying connection system to achieve cost competitiveness compared to conventional generation technologies. For example, larger turbines located farther offshore are more difficult to access. Further, accessibility of offshore assets depends on weather conditions, which can have a significant impact on income and expenditure. Consequently, the operator does not always possess sufficient information to make the most cost-effective decisions relating to planning and maintaining their assets. Similar problems arise from uncertainty over long-term decisions for investment and integration of offshore wind assets.
Existing modelling methods make simplifying assumptions which unfortunately lead to suboptimal solutions, especially in larger wind farms where many factors are at play. For instance, existing tools typically use two state Markov chains for failure and repair, meaning that failure and repair times are exponentially distributed [1]-[2]. Whilst this can be a reasonable approximation for failure, repair is rarely exponentially distributed due to sometimes large and random lead times to reach the assets depending on weather conditions. Durham has experience with more accurately representing failure and repair processes and handling modelling assumptions, especially under severe uncertainty in the planning stage [3]. The aim of this project is to develop new methods for modelling and optimising decisions involving planning and operation for larger offshore wind farms, especially when facing uncertainties in the available actionable information. We also aim to link failure and repair to the environmental conditions of the wind farm, and operational conditions of individual turbines. We expect this to improve the predictive capability of failures and repairs, including the consideration of turbine accessibility, thereby reducing costs.
The outcome of this project will inform decision-making processes for years to come for efficient integration and operation of larger offshore wind farms paving the way for future developments in a more robust and cost-effective manner.
Methodology
The researcher will develop generalised models for modelling failure/repair processes in offshore wind farm assets, moving away from simplified exponential transition times, and based on existing offshore wind farm operational and sensory data (e.g., SCADA and other CMS data). This data will be made available to the student through access to existing Durham data repositories (e.g., Reliawind Database for SCADA data or HOME-Offshore reliability data – see [4]) as well as Durham’s using existing links with offshore wind developers for access to more current data. This will enable the development of more accurate models that can better capture correlations (and if existing, any causal relationships) between failures and their wider environmental and operational conditions. Simultaneously, this will also inform improved repair practices that are contingent on environmental conditions. Meanwhile, the candidate will use the newly developed failure/repair process models to simulate the operation of exemplar offshore wind farms with a variety of options for offshore wind transmission. In evaluating any metrics of interests (e.g., cost of energy), the candidate will consider uncertainties (either due to inherent variability in the model, or due to limitations in the data) for accurate prediction of wind farm performance metrics. This will help wind farm planners make risk-informed decisions on the choices of their assets for future offshore wind farms.
References & Further Reading
[1] Billinton, R., and Alan, R. (1992), Reliability Evaluation of Engineering Systems, 2nd Edition, Springer, Boston, MA, USA
[2] Li, W. (2014), Risk Assessment of Power Systems: Models, Methods, and Applications, 2nd Edition, Wiley-IEEE
[3] Bains, Henna and Madariaga, Ander and Troffaes, Matthias C. M. and Kazemtabrizi, Behzad (2020) ‘An economic model for offshore transmission asset planning under severe uncertainty’, Renewable energy., 160 . pp. 1174-1184.
[4] Dao, Cuong D., Kazemtabrizi, Behzad & Crabtree, Christopher J. (2019) ‘Wind Turbine Reliability Data Review and Impacts on Levelised Cost of Energy’, Wind Energy WE-19-0119
[5] Bains, Henna and Madariaga, Ander and Kazemtabrizi, Behzad and Troffaes, Matthias C. M. (2019) ‘The impact of offshore transmission regulatory regimes on technology choices.’, in Proceedings of the Cigre Symposium Aalborg, 2019.
[6] Dao, Cuong D. and Kazemtabrizi, Behzad and Crabtree, Christopher J. and Tavner, Peter J. (2021) ‘Integrated condition-based maintenance modelling and optimisation for offshore wind farms.’, Wind Energy 2021, pp. 1-19.