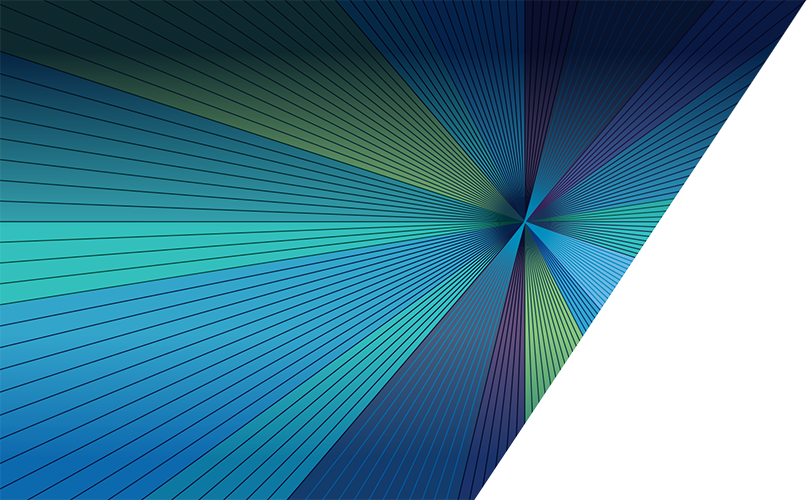
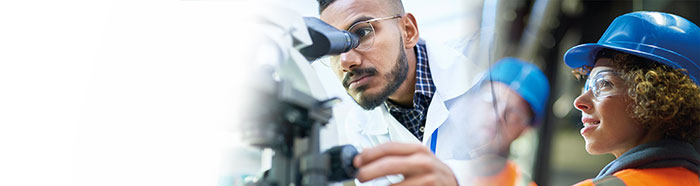
Research projects
- Research area
Push the Frontiers of Offshore Wind Technology
- Institution
University of Hull
- Research project
SafeML-based Confidence Generation and Explainability for UAV-based Anomality Detection of Blades Surface in Offshore Wind Turbines
- Lead supervisor
- PhD Student
- Supervisory Team
Dr Koorosh Aslansefat (Lecturer/Assistant Professor - Faculty of Science and Engineering, University of Hull, University of Hull)
Professor Yiannis Papadopoulos (Professor – Faculty of Science and Engineering, University of Hull)
Project Description:
This PhD scholarship is offered by the EPSRC CDT in Offshore Wind Energy Sustainability and Resilience; a partnership between the Universities of Durham, Hull, Loughborough and Sheffield. The project is part of a Research Cluster focussing on Reliability and Health Monitoring. The successful applicant will undertake six-month of training with the rest of the CDT cohort at the University of Hull before continuing their PhD research at Hull.
Unmanned Aerial Vehicle (UAV) e.g., drones are increasingly used for equipment anomaly and fault detection. When the drones are employed to take images, the quality of the images can be affected by several factors. For instance, images can be blurred due to the relative motion between the blades and the camera mounted on the drones. Noise can be introduced to the images due to the harsh operating conditions of drones. Noise can also be produced by various surrounding electronic devices. As a result, the decision made based on these images could be affected depending on the quality of the images. For this reason, the aim of this project is to propose a methodology to generate confidence in such decision.
Methodology
Figure 1 illustrates the proposed methodology. In this method, the images taken by each drone will be loaded into the pre-processing unit and then the pre-processed data will be used as the input of the deep learning algorithm. In the next phase, the SafeML tool (a novel open-source safety monitoring tool) [1-3] is used to measure the statistical difference between new images and the trusted datasets (the datasets that the deep learning model has been trained with and validated by an expert in the design time) to generate the confidence. Having generated the confidence, three scenarios have been considered; (a) if the confidence is very low, then the approach will provide notice for O&M team to do the manual inspection, (b) if the confidence is low, the approach will ask the drone to take more picture from that specific area, and (c) if the confidence is high, the approach will generate the diagnosis report with addressing the evaluated confidence. In the last scenario, the system will be permitted to proceed with the results autonomously. Note that the threshold defining for the confidence should be tuned in the design time by an expert.
The approach is also capable of providing deep learning explainability and interpretability. To highlight this feature, we give an example. Consider based on drone images, the system diagnoses wrongly a problem like erosion, fatigue, etc and send the maintenance team to fix the problem or at least do a further investigation which is costly for an offshore wind turbine. Three different questions can be considered for three different perspectives; (a) as the owner of the offshore wind, one may want to know why the system made the wrong decision? (b) as the designer of the deep learning-based diagnosis system, one may want to know which part of the algorithm is responsible for the wrong decision (or where is the deep neural network attention)? and as the (c) drone’s third-party company owner, one may want to know what the problem with images, or their pre-processing was that cause the wrong decision. The statistical analysis provided by this approach can explain these kinds of questions.
Figure 1. SafeML concept for confidence measure and explainability for UAV-based Anomality Detection of Blades Surface in Offshore Wind Turbines.
References & Further Reading
[1] Aslansefat, K., Sorokos, I., Whiting, D., Kolagari, R. T., & Papadopoulos, Y. (2020, September). SafeML: Safety Monitoring of Machine Learning Classifiers Through Statistical Difference Measures. In International Symposium on Model-Based Safety and Assessment (pp. 197-211). Springer, Cham.
[2] Aslansefat, K., Kabir, S., Abdullatif, A., Vasudevan, V., & Papadopoulos, Y. (2021). Toward Improving Confidence in Autonomous Vehicle Software: A Study on Traffic Sign Recognition Systems. Computer, 54(8), 66-76.
[3] The SafeML projects, tools, dataset, and related papers, https://github.com/ISorokos/SafeML.
Training and development
You will benefit from a taught programme, giving you a broad understanding of the breadth and depth of current and emerging offshore wind sector needs. This begins with an intensive six-month programme at the University of Hull for the new student intake, drawing on the expertise and facilities of all four academic partners. It is supplemented by Continuing Professional Development (CPD), which is embedded throughout your 4-year research scholarship.
Entry requirements
If you have received a First-class Honours degree, or a 2:1 Honours degree and a Masters, or a Distinction at Masters level with any undergraduate degree (or the international equivalents) in engineering, computer science or mathematics and statistics, we would like to hear from you.
If your first language is not English, or you require Tier 4 student visa to study, you will be required to provide evidence of your English language proficiency level that meets the requirements of the Aura CDT’s academic partners. This course requires academic IELTS 7.0 overall, with no less than 6.0 in each skill.
The deadline for applications is Wednesday 4 December 2024.
If you have any queries about this project, please contact Dr. Zhibao Mian, z.mian2@hull.ac.uk
You may also address queries about the CDT to auracdt@hull.ac.uk.
Watch our short video to hear from Aura CDT students, academics and industry partners:
Funding notes
The CDT is funded by the EPSRC, allowing us to provide scholarships that cover fees plus a stipend set at the UKRI nationally agreed rates. These are currently circa £19,795 per annum at 2025/26 rates and will increase in line with the EPSRC guidelines for the subsequent years (subject to progress).
Eligibility
Research Council funding for postgraduate research has residence requirements. Our CDT scholarships are available to Home (UK) Students. To be considered a Home student, and therefore eligible for a full award, a student must have no restrictions on how long they can stay in the UK and have been ordinarily resident in the UK for at least 3 years prior to the start of the scholarship (with some further constraint regarding residence for education). For full eligibility information, please refer to the EPSRC website.
We also allocate a number of scholarships for International Students per cohort.
How to apply
Recruitment is open until Wednesday 4 December 2024 for CDT Scholarships beginning study in September 2025.
Please note, you may only apply for ONE project offered through the EPSRC CDT in Offshore Wind Energy Sustainability and Resilience.
Please ensure that you familiarise yourself with the Aura CDT website before you apply to give you a good understanding of what a CDT is, our CDT’s research focus and the training and continuing professional development programme that runs alongside the CDT. The Frequently asked questions page and Candidate resources page are essential reading prior to applying.
Applications to this project are made via the University of Hull admissions system. If you have not applied to the University of Hull before, you will need to set up an account to enable you to track the progress of your application and upload supporting documents.
Follow this link to apply for this CDT project at the University of Hull:
With your application, you need to upload copies of the following supporting evidence:
- Complete transcripts (and final degree certificate(s) where possible). If your qualification documents are not in English, you will need to supply copies of your original language documents as well as their official translation into English.
- Your Curriculum Vitae (CV).
- A completed Supplementary Application Form (upload when asked to add a Research proposal).
Uploading the form
When you have completed the form, please save it as a pdf format and labelled as follows:
Last name_first name PhD application form
Upload the form as part of your application documents through the University of Hull student application portal, when asked to add a Research Proposal. The Form replaces the Research Proposal and so you do not need to add a Research Proposal. Please do not send your form directly to the Offshore Wind CDT.
Guaranteed interview scheme
The CDT is committed to generating a diverse and inclusive training programme and is looking to attract applicants from all backgrounds. We offer a Guaranteed Interview Scheme for home fee status candidates who identify as Black or Black mixed or Asian or Asian mixed if they meet the programme entry requirements. This positive action is to support recruitment of these under-represented ethnic groups to our programme and is an opt in process. Find out more.
Interviews
Interviews will be held during mid-January 2025.