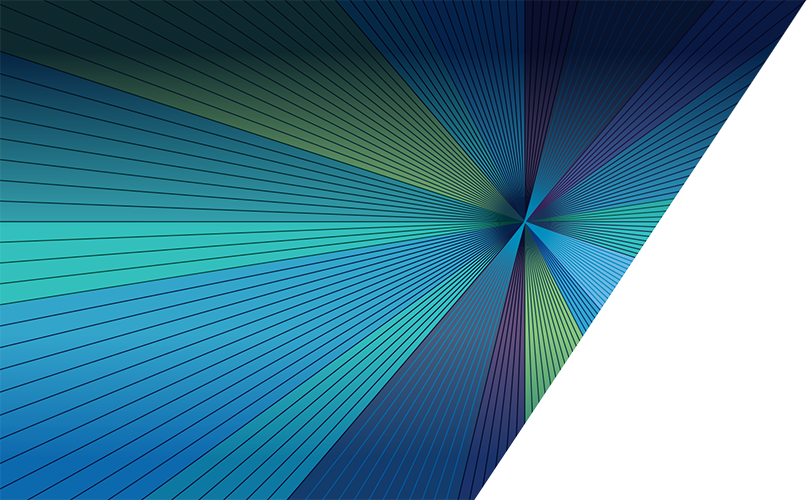
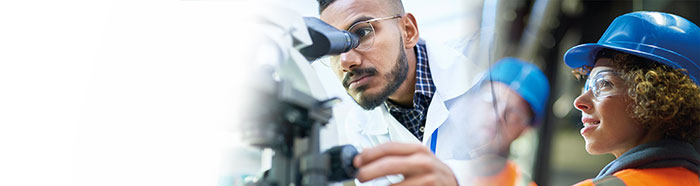
Research projects
- Research area
Build and support a sustainable workforce
- Institution
University of Hull
- Research project
A Holistic Predictive Maintenance Framework for Offshore Wind Turbines Components
- Lead supervisor
Dr Zhibao Mian (Lecturer - Faculty of Science and Engineering, University of Hull)
- PhD Student
- Supervisory Team
Dr Koorosh Aslansefat (Lecturer/Assistant Professor - Faculty of Science and Engineering, University of Hull)
Prof Nina Dethlefs (Professor of Computer Science (Artificial Intelligence), Loughborough University)
Project Description:
This Research Project is part of the Aura CDT’s Maintenance Cluster.
Operation and maintenance (O&M) of offshore wind turbines is inherently a challenging and costly task. Offshore wind turbines are composed of different equipment components, and the fault diagnosis models suitable for each equipment component are also different. The offshore wind industry requires the construction of an effective mechanism that can dynamically unify the components of the entire wind turbines with its applicable diagnostic models.
The current efforts in maintenance for offshore wind farm have been moving from condition-based maintenance [1] towards predictive maintenance approaches [2-5]. These predictive approaches use operational data and AI i.e. Machine Learning (ML) for failure diagnosis, prediction, and prognosis. Academic survey [2] has identified that pitch, gearbox, generator, and blades are most likely to fail and thus these are the components where the most effort has been placed in terms of failure prediction and prognosis.
These efforts, however, mainly focus on single component predictions and prognosis. A step-change is needed, allowing the integration of key components together into a holistic wind turbine model so that it could predict failures across key components and display results at both component and wind turbine level. Similarly, current AI predictive models are often designed for use at one site, based on unique site-specific parameters. This limits the scalability, applicability, and utility of the models.
The objective of this project is to explore ways to build a dynamic and holistic wind turbine predictive maintenance model adaptable for different components and sites. For any type of wind turbines, the proposed method should dynamically call the diagnosis and prediction model according to different types and conditions of its component equipment to achieve automated and accurate prediction and diagnosis. In addition, considering the generality of the method, it should be able to dynamically provide optimal prediction and maintenance solutions based on equipment type and working environment.
Methodology
Based on emerging AI methodologies, the project will study various AI-based failure diagnosis and prediction models and approaches that could be used for key components of wind turbine [2-5]. A comparison will be made of the performance of these models, establishing a comprehensive evaluation system to fairly justify the performance and feasibility from the perspective of offshore wind turbines. Furthermore, the project will design and develop a novel holistic failure diagnosis and prediction framework for different components under different operation environments. In this framework, a predictive model generator [3] would be used for generating different predictive models for different monitored wind turbines.
References & Further Reading
[1] Tchakoua, P.; Wamkeue, R.; Ouhrouche, M.; Slaoui-Hasnaoui, F.; Tameghe, T.A.; Ekemb, G. Wind Tur- bine Condition Monitoring: State-of-the-Art Review, New Trends, and Future Challenges. Energies 2014, 7, 2595-2630. https://doi.org/10.3390/en7042595.
[2] Fox, H.; Pillai, A.C.; Friedrich, D.; Collu, M.; Da- wood, T.; Johanning, L. A Review of Predictive and Prescriptive Offshore Wind Farm Operation and Maintenance. Energies 2022, 15, 504. https://doi.org/10.3390/en15020504
[3] M. Canizo, E. Onieva, A. Conde,S. Charramendieta and S. Trujillo, ”Real-time predictive maintenance for wind turbines using Big Data frame- works,” 2017 IEEE International Conference on Prognostics and Health Management (ICPHM), 2017, pp. 70-77, doi: 10.1109/ICPHM.2017.7998308.