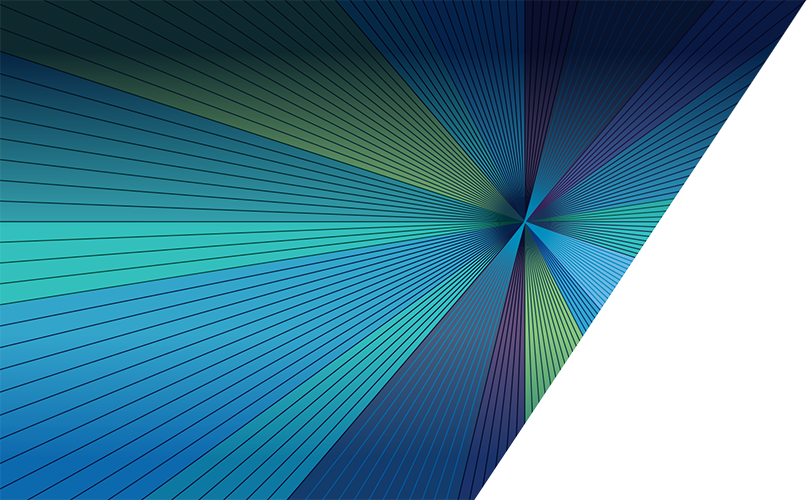
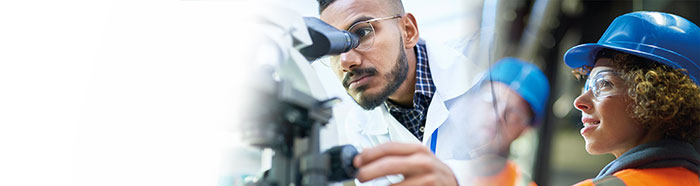
Research projects
- Research area
Operations maintenance and human factors
- Institution
University of Sheffield
- Research project
Advanced data analysis and robust statistics for structural health monitoring of wind turbines: from individual components to a fleet
- Lead supervisor
Prof Nikolaos Dervilis (Professor of Mechanical Engineering, University of Sheffield)
- PhD Student
- Supervisory Team
Professor Keith Worden (Professor of Mechanical Engineering, University of Sheffield)
Project Description:
As offshore wind turbines and engineering systems are entering a new technological era; more sensing systems are adopted which results to a geometrical increase of high sampling data. Especially, data that is concerned with the health monitoring of these structures is increasing geometrically as it needs to look not only to individual components but to the healthy performance of the fleet as well.
This project aims to promptly process the massive collection of data and automatically provide advanced data features that are rich in information not only for wind farms but for engineering structures in general as well. Machine leaning tools like deep learning architectures and robust statistics shall be investigated spanning from deep neural networks to Gaussians Processes (GPs) and robust outlier analysis and regression. The aim is to provide robust processing of data in order to deliver intelligent data understanding across individual structural components and populations of wind turbine fleets and farms (e.g. informative structural health diagnosis systems (SHM) under various operating and environmental conditions) so, that inferences across structures, features and active learning can be obtained.
Methodology
It will be necessary to develop Bayesian and deep learning architectures and tools which process the collection of data and automatically provide advanced data features that are rich in information, through time and the evolution of the structure. This will make use of tools like active [2], semi-supervised and transfer learning. To build such models this project will make use of the latest developments in machine learning and statistics, in particular, techniques like Gaussians Processes (GPs), Transfer learning and Adaptive Neural Networks will be considered in order to create populations of structural inferences. Advanced and robust statistics like outlier analysis and immune to outliers regression will also be considered.
By combining these modern state of the art tools it will be possible to develop approaches to provide online, fast and sparse processing of huge amounts of data in order to deliver intelligent data understanding, including their associated uncertainty in their predictions. Data will be generated from low frequencies (modal testing) to ultrasonic guided waves within the Dynamics Research Group (DRG) facilities. The environmental chambers and testing via the Laboratory for Verification and Validation (LVV) will be used to explore scenarios relating to SHM under environmental and operational valuations via the advanced experimental environmental champers. Further, the DRG group and the supervisor team have parallel projects in offshore wind with industrial partners that will be used to create links for advice and data.