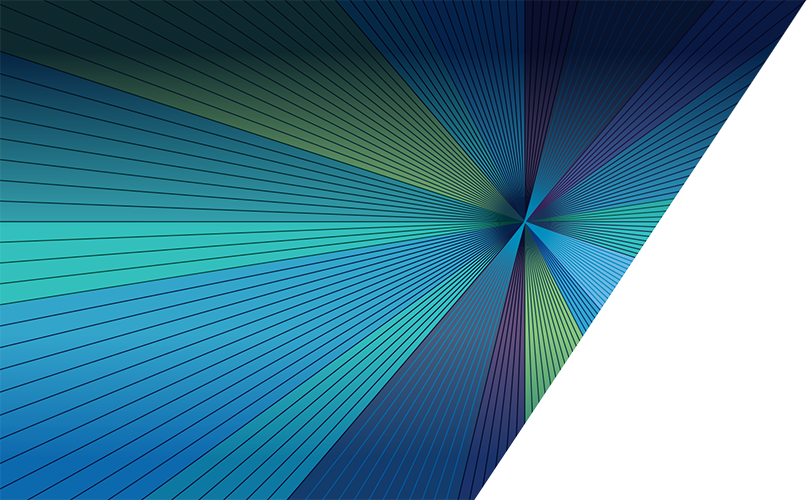
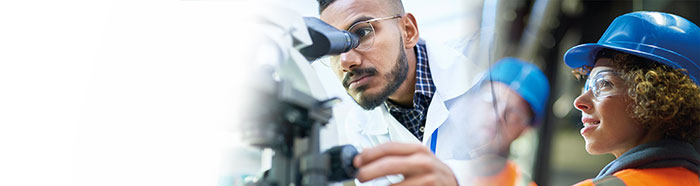
Research projects
- Research area
Build and support a sustainable workforce
- Institution
University of Hull
- Research project
An RCM-based Intelligent Maintenance Framework for Offshore Wind Turbines
- Lead supervisor
Dr Zhibao Mian (Lecturer - Faculty of Science and Engineering, University of Hull)
- PhD Student
- Supervisory Team
Professor Yiannis Papadopoulos (Professor – Faculty of Science and Engineering, University of Hull)
Project Description:
This Research Project is part of the Aura CDT’s Maintenance Cluster.
Operations and Maintenance (O&M) costs are a significant element in offshore wind farm budgets, therefore reducing O&M costs can have a major impact on reducing energy costs to consumers.
The development of appropriate and efficient maintenance strategies to maintain higher reliability and availability of wind turbines with lower maintenance costs remains one of the core tasks of operations and maintenance (O&M). The principle of RCM (Reliability Centric Maintenance) can be applied to reach a balance between O&M costs and reliability for wind turbines [1].
It is becoming clear that predictive maintenance could improve reliability, reduce downtime, helping to reduce overall O&M costs in the wind industry [2]. It is estimated that the costs of O&M in offshore wind farms could be significantly reduced through RCM-based data-driven predictive maintenance. This requires exploring how RCM could be integrated with the latest predictive maintenance models in the context of intelligent maintenance.
Embedding RCM technology in intelligent maintenance requires the designing of a new interface, where offline RCM failure data and online operation and maintenance data are transformed and integrated into an AI-based e-maintenance system. This interface would need to allow for the selection of appropriate predictive maintenance models for specific wind turbines.
The aim of this project is to explore feasible solutions to address these challenges by developing a novel framework for integrating RCM with predictive maintenance.
Methodology
The RCM-based, data-driven, intelligent maintenance framework could be started with a model-based RCM analysis [3-4] to automatically determinise an optimal maintenance strategy (e.g., reactive maintenance, preventive maintenance, and predictive maintenance) that should be applied to each key component of wind turbine according to maintenance and cost requirements.
Then, if a predictive maintenance strategy is determined, an architecture should be created through a consideration of the data that are available from the various online and offline sources, and how this could be used to support the development of an intelligent maintenance system [2].
A promising architecture could combine RCM technology with state-of-the-art deep machine learnings and model-based reliability analysis technologies to provide an advanced real-time data-driven intelligent maintenance framework. The benefit of this framework is that it drives the rapid evolution of maintenance to digitalisation, automation, and intelligence.
References & Further Reading
[1] Pattison, D., Segovia Garcia, M., Xie, W., Quail, F., Revie, M., Whitfield, R.I. and Irvine, I., 2016. Intelli- gent integrated maintenance for wind power generation. Wind Energy, 19(3), pp.547-562.
[2] Fox, H.; Pillai, A.C.; Friedrich, D.; Collu, M.; Dawood, T.; Johanning, L. A Review of Predictive and Prescriptive Offshore Wind Farm Operation and Maintenance. Energies 2022, 15, 504. https://doi.org/10.3390/en15020504
[3] Z. Mian, S. Jia, X. Shi, C. Tang, J. Chen, and Y. Gao, ”A Model-based RCM Analysis Method,” in 2020 IEEE 20th International Conference on Software Quality, Reliability and Security Companion (QRS-C), 2020, pp. 301-307.
[4] Fischer, K.; Besnard, F.; Bertling, L. ”Reliability-Centered Maintenance for Wind Turbines Based on Statistical Analysis and Practical Experience,” IEEE Transactions on Energy Conversion, 2012, vol 27, pp.1-12, doi:10.1109/TEC.2011.2176129.