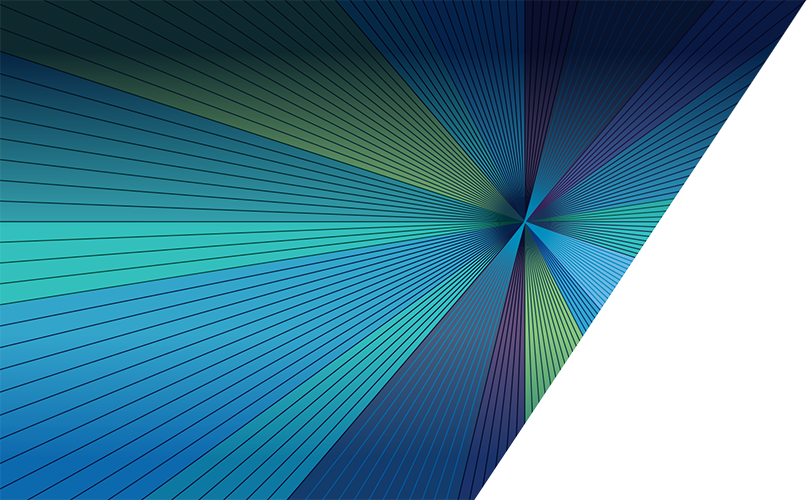
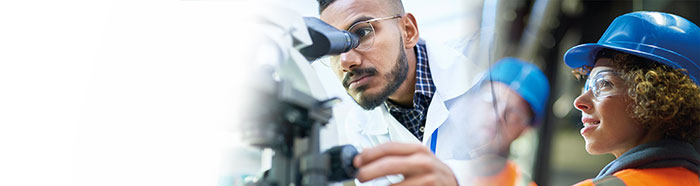
Research projects
- Research area
Big data sensors and digitalisation for the offshore environment
- Institution
University of Hull
- Research project
DIAS: Data-driven Intelligent Alarm System
- Lead supervisor
Professor Yiannis Papadopoulos (Professor – Faculty of Science and Engineering, University of Hull)
- PhD Student
- Supervisory Team
Professor James Gilbert (Director - Energy & Environment Institute, University of Hull)
Dr Umar Manzoor
Project Description:
We propose to develop an advanced alarm processing system for wind turbines using data analytics and machine learning. The system will be able to filter false alarms, synthesise low level alarms and localise faults from a plethora of low-level sensor data. Sensors in wind farms monitor equipment or environmental conditions. Using them, patterns of abnormal behaviours will be detected via machine learning techniques and alarms and relevant procedures will be generated. This is vital for safety and efficiency. Typically, a large number of data makes the process of fault localisation difficult. This is confusing operators, reduces efficiency of maintenance and increases costs, a problem that project DIAS will address. The PhD will build on our recent pioneering work on improving the accuracy of machine learning via statistical detection of distributional shifts between data used to train algorithms and data encountered during operation. Results of the PhD will have useful application in other domains, for example medical diagnosis, as well as abnormal event detection in robotics and autonomous systems, i.e. all areas where machine learning classifiers would benefit from greater accuracy.
Methodology
DIAS will be undertaken within the Dependable Intelligent Systems (DEIS) group in collaboration with University of Hull Engineering staff and staff at EDF that will provide domain knowledge on OSW and know-how on data-sets. Data-driven diagnosis and prognosis using machine learning and statistical techniques will be combined in a model to achieve the project goals. The training of machine learning algorithms will be done using 80% of the data provided by EDF already pointing to causal relationships between abnormalities, alarms and actions. Algorithms will be tested on the remaining 20% of data. Assuming accuracy, we will also strive to achieve live application of this system, as advisory tool, in an EDF facility. Our work on improving the accuracy of machine learning will be used to continually inform operators about the trustworthiness of the algorithms as operation evolves. We note that the DEIS research group pioneers advanced methods and state-of-the-art commercial tools for the design of dependable intelligent systems. Commercial tools include HiP-HOPS and Safety Designer. We also lead new AI algorithms which imitate the hunting behaviour of Penguins, work that has received high profile publicity (see, for example this BBC article on “Hungry Penguins Keep Car Code Safe“).
References & Further Reading
1. Aslansefat K., Sorokos I., Papadopoulos I. (2020) SafeML: Safety Monitoring of Machine Learning Classifiers Through Statistical Difference Measures, Int’l Symposium on Model-based Safety Assessment, Springer Lecture Notes in CS vol. 12297: pp. 197-211
2. Gheraibia, Y., Kabir, S., Aslansefat, K., Sorokos, I., & Papadopoulos, Y. (2019) Safety+ AI: A Novel Approach to Update Safety Models Using Artificial Intelligence. IEEE Access, DOI: 10.1109/ACCESS.2019.2941566, IEEE
3. Aizpurua J, Papadopoulos Y., Merle G. (2018) Explicit Modelling and Treatment of Repair in Prediction of Dependability, IEEE Transactions on Dependable and Secure Computing, DOI: 10.1109/TDSC.2018.2857810, IEEE
4. Papadopoulos Y., Walker M., Parker D., Sharvia S., Bottaci L., Kabir S., Azevedo L., Sorokos I. (2016) A Synthesis of Logic and Bio-inspired techniques in the Design of Dependable Systems, Annual Reviews in Control, 41: 170-182, IFAC & Elsevier