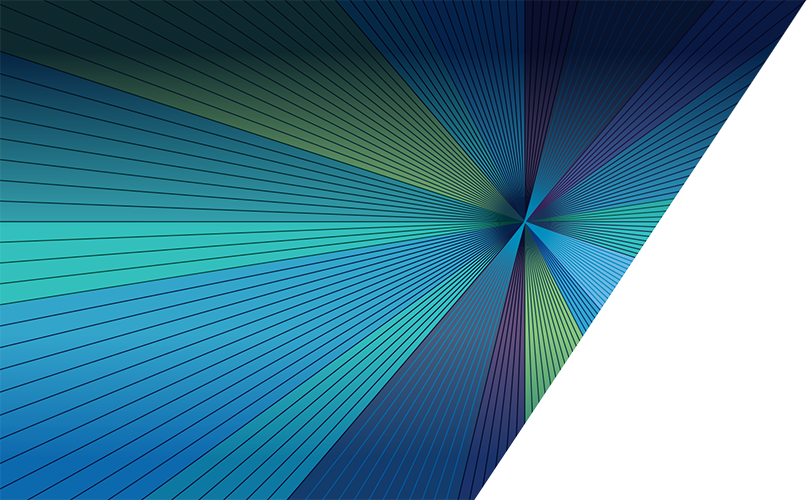
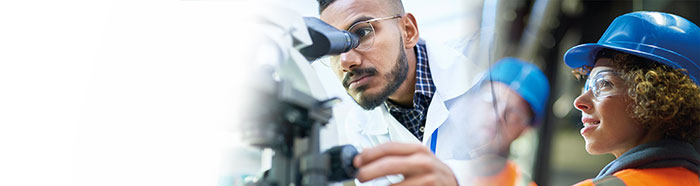
Research projects
- Research area
Achieve a sustainable wind farm life cycle
- Institution
University of Sheffield
- Research project
Digital twins for health monitoring and fault detection in wind generators and converters
- Lead supervisor
Professor Antonio Griffo (Professor – Electrical Engineering, University of Sheffield)
- PhD Student
- Supervisory Team
Dr Xiao Chen (Lecturer in Electrical Machines - Department of Electronic and Electrical Engineering, University of Sheffield)
Project Description:
This PhD scholarship is offered by the EPSRC CDT in Offshore Wind Energy Sustainability and Resilience, a partnership between the Universities of Durham, Hull, Loughborough and Sheffield. The successful applicant will undertake six-month of training with the rest of the CDT cohort at the University of Hull before continuing their PhD research at the University of Sheffield. The project is part of a Research Cluster focusing on Reliability and Health Monitoring.
Reliability is of paramount importance for the offshore wind industry as the cost of maintenance, downtime and repair can markedly affect the business case for adopting new and innovative technologies.
To increase availability without increasing maintenance and associated downtime, condition and health monitoring to support fault detection and predictive maintenance are essential in offshore wind. Although many CHM tools are being investigated for the structural elements of a wind generator, little has been done for the electrical generators and power electronics converters which are at the heart of the energy conversion system.
The digital twin concept, based on an accurate real-time simulation of the real system, has emerged as a powerful tool for condition monitoring and predictive maintenance.
Digital twin concepts are gaining interest in the research and industrial communities as effective tools to support smart manufacturing, operation and monitoring of industrial processes and equipment.
Digital twins (DT) are multiphysics, multiscale, high-fidelity simulation that emulate in real-time the state of a corresponding physical twin based on historical and real-time sensors data. The comparison of physical and virtual data throughout product lifecycle can provide valuable information on the state-of-health of a physical structure. While extensive research is being undertaken on DTs for structural health monitoring in OW, there is little if any application of the DT concept to electrical equipment. This is mainly due to the difficulties of multi-time scale modelling in the electrical domain where dynamics can range from sub-milliseconds transients following a power electronics switching transient to thermal and mechanical induced gradual ageing and degradation taking place over the lifetime of the machine.
Using a combination of high-fidelity analytical models, model order reduction techniques and machine learning, this project will develop and validate a multi-time scale digital twin concept for advanced condition monitoring and maintenance of direct-drive permanent magnet generators and converters for offshore wind. The proposed digital twin, will be able to accurately model all electrical transients in the electric drive train, ranging from the sub-millisecond time-scale of the switching converter to long-term degradation over the lifetime of the machine. Comparison of the digital-twin output and the real-time measurements from a range of sensors, combined with advanced signal-processing tools, will be used to demonstrate the ability to detect both gradual degradation and faults in the machine.
Training and development
You will benefit from a taught programme, giving you a broad understanding of the breadth and depth of current and emerging offshore wind sector needs. This begins with an intensive six-month programme at the University of Hull for the new student intake, drawing on the expertise and facilities of all four academic partners. It is supplemented by Continuing Professional Development (CPD), which is embedded throughout your 4-year research scholarship.
Entry requirements
If you have received a First-class Honours degree, or a 2:1 Honours degree and a Masters, or a Distinction at Masters level with any undergraduate degree (or the international equivalents) in engineering, mathematics and statistics or physics, we would like to hear from you.
If you have any queries about this project, please contact Prof Antonio Griffo, a.griffo@sheffield.ac.uk
You may also address queries about the CDT to auracdt@hull.ac.uk.
Watch our short video to hear from Aura CDT students, academics and industry partners:
Funding
The CDT is funded by the EPSRC, allowing us to provide scholarships that cover fees plus a stipend set at the UKRI nationally agreed rates. These have been set by UKRI as £20,780 per annum at 2025/26 rates and will increase in line with the EPSRC guidelines for the subsequent years (subject to progress).
Eligibility
Our funded Doctoral Scholarships are available to UK Students. The advertised CDT scholarships in this current recruitment round are available to Home (UK) Students only as the CDT has reached the annual cap, set by the funding council (UKRI EPSRC), on international student recruitment for the 2025 intake. To be considered a Home student, and therefore eligible for a full award, a student must have no restrictions on how long they can stay in the UK and have been ordinarily resident in the UK for at least 3 years prior to the start of the scholarship (with some further constraint regarding residence for education).
Guaranteed Interview Scheme
The CDT is committed to generating a diverse and inclusive training programme and is looking to attract applicants from all backgrounds. We offer a Guaranteed Interview Scheme for home fee status candidates who identify as Black or Black mixed or Asian or Asian mixed if they meet the programme entry requirements. This positive action is to support recruitment of these under-represented ethnic groups to our programme and is an opt in process.
How to apply
Recruitment has closed for this project and applications are being assessed for September 2025 entry.
Interviews will be held online with an interview panel comprising of project supervisory team members from the host university where the project is based. Where the project involves external supervisors from university partners or industry sponsors then representatives from these partners may form part of the interview panel and your supplementary application form will be shared with them (with the guaranteed interview scheme section removed). Interviews will take place during early and mid-June.
If you have any queries about this project, please contact Prof Antonio Griffo, a.griffo@sheffield.ac.uk
You may also address queries about the CDT to auracdt@hull.ac.uk.