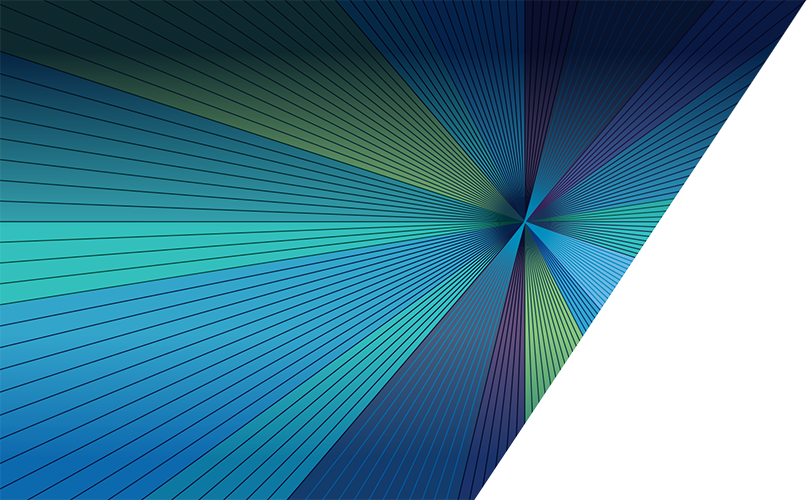
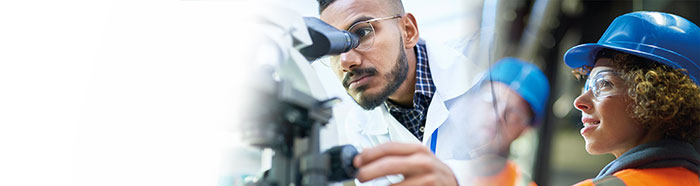
Research projects
- Research area
Push the Frontiers of Offshore Wind Technology
- Institution
Durham University
- Research project
Optimisation of wind farms power production using AI
- Lead supervisor
Dr Stefano Giani (Assistant Professor in the Department of Engineering, Durham University)
- PhD Student
- Supervisory Team
Dr Majid Bastankhah (Associate Professor, Department of Engineering, Durham University)
Marc Deisenroth (The Alan Turing Institute)
Project Description:
This PhD scholarship is offered by the EPSRC CDT in Offshore Wind Energy Sustainability and Resilience; a partnership between the Universities of Durham, Hull, Loughborough and Sheffield. The project is sponsored by industry partner, The Alan Turing Institute. The successful applicant will undertake six-month of training with the rest of the CDT cohort at the University of Hull before continuing their PhD research at Durham University.
Wind power contributed 12% of UK electricity demand in 2019. This placed the United Kingdom at this time as the world’s sixth-largest producer of wind power. The efficiency of wind farms is of vital importance for the UK to rely on wind power.
Wind turbines are very efficient in a steady airflow. These ideal conditions are rarely met in real wind farms because of turbine wakes. Significant research over the past two decades has highlighted the flow physics within turbine wakes as the main source of power losses in wind farms. Turbine wakes are characterised by low-energy and highly turbulent regions, which form downwind of wind turbines. Due to turbine wakes, whose effects, in the right conditions, can last over a few kilometres, there is less energy available for downstream wind turbines.
Prior research has shown that wind farm power losses due to turbine wakes alone can, depending on atmospheric conditions and the siting of the turbine with respect to the incoming wind, exceed 40%. Moreover, the high turbulence within the wake flows increases the unsteady structural loads on wind turbines, and thereby reduces their lifetimes. For these reasons, wind-energy professionals use sophisticated numerical and experimental techniques to predict turbine wakes and examine ways to mitigate their effects on wind farm performance. This is given that even a 1% reduction in the LCOE through wake flow mitigation strategies (e.g., wake steering) will translate into millions of pounds of revenue each year.
However, many different scenarios need to be tested due to the continuous change in real-life operating conditions (e.g., change in wind speed, wind incidence, atmospheric thermal stability). This means that wind farm designers rarely have the luxury to afford the sophisticated techniques used in the research community due either to a lack of funding or time constrains. For this reason, this project aims at delivering a novel artificial intelligence (AI) capable to optimise the control parameters of a wind farm in response to changing wind conditions and to power demand from the grid.
Methodology
This project will develop an artificial intelligence (AI) capable to optimise the control parameters of a wind farm in response to changing wind conditions and to power demand from the grid. We propose to use Deep Reinforcement Learning (DRL) to train an AI capable to control virtual wind farms. DRL has been used to solve main AI challenges like in the case of AlphaGo beating the world champion of Go.
We will also consider a model-based RL option, where a machine learning serves as a surrogate model and then used to determine optimal control strategies. Here, we have the opportunity to train the model on real-world data.
An alternative approach to optimisation of control parameters of a wind farm is Bayesian optimisation, a data-driven Bayesian optimisation technique that can be used to efficiently optimise a small number of critical parameters (it’s also been used to optimise meta-parameters, such as step sizes, in deep learning settings).
Training and development
You will benefit from a taught programme, giving you a broad understanding of the breadth and depth of current and emerging offshore wind sector needs. This begins with an intensive six-month programme at the University of Hull for the new student intake, drawing on the expertise and facilities of all four academic partners. It is supplemented by Continuing Professional Development (CPD), which is embedded throughout your 4-year research scholarship.
During the project, you will learn state of the art of AI techniques that can be applied in a variety of fields. You will also work on a very important engineering problem that could have a big impact on the future of energy production from wind. After the successful completion of the proposed project, you could either pursue a career in academia or the renewable energy private sector.
Entry requirements
If you have received a First-class Honours degree, or a 2:1 Honours degree and a Masters, or a Distinction at Masters level with any undergraduate degree (or the international equivalents) in engineering, computer science, mathematics and statistics, physics, we would like to hear from you.
If your first language is not English, or you require Tier 4 student visa to study, you will be required to provide evidence of your English language proficiency level that meets the requirements of the CDT’s academic partners. This course requires academic IELTS 7.0 overall, with no less than 6.0 in each skill.
If you have any queries about this project, please contact Prof Stefano Giani, stefano.giani@durham.ac.uk
You may also address queries about the CDT to auracdt@hull.ac.uk.
Watch our short video to hear from Aura CDT students, academics and industry partners:
Funding
The CDT is funded by the EPSRC, allowing us to provide scholarships that cover fees plus a stipend set at the UKRI nationally agreed rates. These are currently circa £19,795 per annum at 2025/26 rates and will increase in line with the EPSRC guidelines for the subsequent years (subject to progress).
Eligibility
Research Council funding for postgraduate research has residence requirements. Our CDT scholarships are available to Home (UK) Students. To be considered a Home student, and therefore eligible for a full award, a student must have no restrictions on how long they can stay in the UK and have been ordinarily resident in the UK for at least 3 years prior to the start of the scholarship (with some further constraint regarding residence for education). For full eligibility information, please refer to the EPSRC website.
We also allocate a number of scholarships for International Students per cohort.