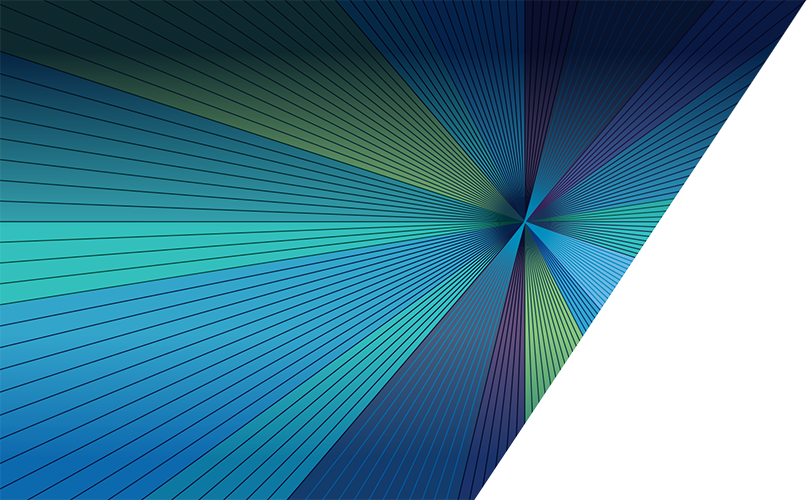
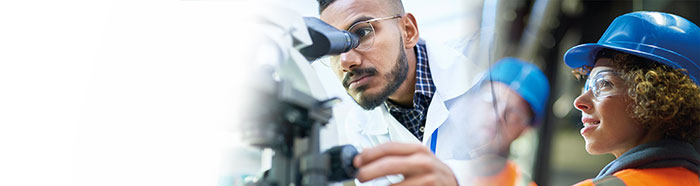
Research projects
- Research area
Big data sensors and digitalisation for the offshore environment
- Institution
University of Sheffield
- Research project
Population-based offshore wind farm fleet health monitoring and performance prediction
- Lead supervisor
Professor Keith Worden (Department of Mechanical Engineering, University of Sheffield)
- PhD Student
- Supervisory Team
Prof Nikolaos Dervilis (Professor of Mechanical Engineering, University of Sheffield)
Dr L.A Bull, Research Associate, Alan Turing Institute
Project Description:
As offshore wind turbines and engineering systems are entering a new technological era, more sensing systems are adopted, which results in a geometrical increase of high-sampled data. This project aims to promptly process this massive collection of data and automatically provide advanced data features that are rich in information, not only for wind farms but also for engineering structures in general. Operations and maintenance of OSW is a key expenditure within the industry, this project directly addresses an approach for managing those cost. It focusses on improved early detection and quantification of defects on wind turbines and sparse data. The project will support the sector with online preventative maintenance, safety and provide increased lifetime extension.
Offshore wind farms are entering a new era; more sensing systems are adopted, which results in a geometrical increase of high-sampled data. Europe is presenting a rapidly growing interest in exploring and investing in such offshore power plants, as the continent’s water sites offer impressive wind conditions. The cost of an offshore wind farm is relatively high, and therefore their reliability is crucial if they ever need to be fully integrated into the energy arena. Until recently, the way the research community and industry have addressed the monitoring problem has mainly been on an individual structure or component basis. However, it is true to say that monitoring and performance analysis is still facing challenges on this individual basis because of a paucity of damage-state data, missing labels, operational and environmental fluctuations and/or computational costs. Consequently, population-based monitoring is proposed as a means of facing some of these challenges.
Machine leaning tools like deep learning architectures and robust statistics shall be investigated ranging from deep neural networks to Gaussians Processes (GPs) and robust outlier analysis and regression [1-4]. The aim is to provide robust processing of data in order to deliver intelligent data understanding across individual structural components and populations of wind turbine fleets and farms (e.g. informative structural health
diagnosis systems (SHM)) under various operating and environmental conditions) so, that inferences across structures, features and active learning can be obtained [2,5-6]. An advanced and robust machine-learning approach will be applied, in order to produce individual and population-based predictions across farms and then predict measurements from each wind turbine (WT) from the measurements of the other WTs in the farm. Control charts with robust thresholds calculated from extreme-value statistics are successfully applied for the monitoring of the turbines
The project will provide the sector state of the art new technologies in AI and Machine learning for maintenance and monitoring of populations of offshore wind farms and their components as well as tools that will be critical tools on the digital twin era.
The Dynamics Research Group (DRG) is a world leading group in advanced data analysis and dynamics with 14 Academics and over 60 PhD students and postdoctoral researchers. The DRG owns one the most advanced labs in the world the LVV: https://lvv.ac.uk/explore-3d
Methodology
We will develop Bayesian technologies and tools which process the collection of data and automatically provide advanced data features that are rich in information, through time and the evolution of the structure. This project will make use of tools like active [2], semi-supervised and transfer learning [5-6]. To build such models, the project will make use of the latest developments in machine learning and statistics; in particular, techniques like Gaussians Processes (GPs), Furthermore tools like outlier analysis will also be considered [1-3]. We will develop approaches to provide online, fast and sparse processing of data in order to deliver intelligent data understanding, including the associated uncertainty in predictions.
Offshore wind farm SCADA data will be used from the team’s connections with Vattenfall and Siemens-Gamesa. The DRG group and the supervisor team have parallel projects in offshore wind with industrial partners that will be used to create links for advice and data (https://npow.group.shef.ac.uk/). Also the project will utilise data from [4] if needed and if useful for the project.
References & Further Reading
[1] Bull LA, Worden K, Fuentes R, Manson G, Cross EJ, Dervilis N. Outlier ensembles: A robust method for damage detection and unsupervised feature extraction from high-dimensional data. Journal of Sound and Vibration. 2019 Aug 4;453:126-50.
[2] Bull LA, Rogers TJ, Wickramarachchi C, Cross EJ, Worden K, Dervilis N. Probabilistic active learning: An online framework for structural health monitoring. Mechanical Systems and Signal Processing. 2019 Dec 1;134:106294.
[3] Fuentes R, Gardner P, Mineo C, Rogers TJ, Pierce SG, Worden K, Dervilis N, Cross EJ. Autonomous ultrasonic inspection using Bayesian optimisation and robust outlier analysis. Mechanical Systems and Signal Processing. 2020 Nov 1;145:106897.
[4] Papatheou E, Dervilis N, Maguire AE, Antoniadou I, Worden K. A performance monitoring approach for the novel Lillgrund offshore wind farm. IEEE Transactions on Industrial Electronics 2015; 62(10):6636–6644.
[5] Bull LA, Gardner PA, Gosliga J, Rogers TJ, Dervilis N, Cross EJ, Papatheou E, Maguire AE, Campos C, Worden K. Foundations of population-based SHM, Part I: Homogeneous populations and forms. Mechanical Systems and Signal Processing. 2020;148:107141.
[6] Gardner P, Bull LA, Gosliga J, Dervilis N, Worden K. Foundations of population-based SHM, Part III: Heterogeneous populations–Mapping and transfer. Mechanical Systems and Signal Processing.;149:107142.