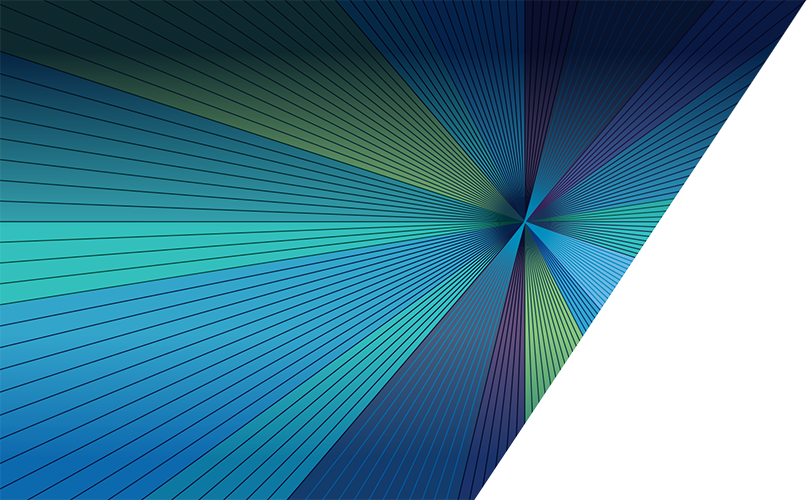
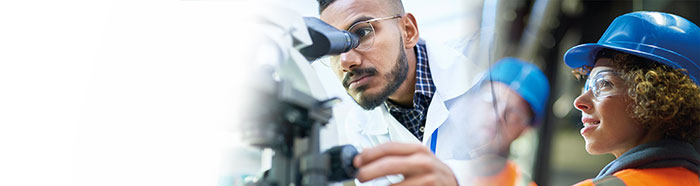
Research projects
- Research area
Big data sensors and digitalisation for the offshore environment
- Institution
University of Sheffield
- Research project
Ultrasonic guided waves blade monitoring
- Lead supervisor
Dr Timothy Rogers (Senior Lecturer in Mechanical Engineering, University of Sheffield)
- PhD Student
- Supervisory Team
Prof Elizabeth Cross, School of Mechanical, Aerospace and Civil Engineering, University of Sheffield
Project Description:
Ultrasonic waves are an excellent tool for assessing damage in structural components and especially composite offshore wind turbine blades materials. By considering changes in pulses of waves through a material, damage or other defects can be detected with very high accuracy. For composite materials, such as those used in wind turbine blades, the propagation of the waves is physically complicated and this can make damage detection difficult. This project will consider how by quantifying uncertainty and applying machine learning to in-service ultrasonic inspection could be used over wider areas, with greater accuracy and sensitivity and provide more robust inspection methods.
This project will consist of a combination of experimental work in the LVV (lvv.ac.uk) collecting data from wind turbine blade samples and data analysis using machine learning. Experiments will consist of arrays of PZT actuators and sensors in combination with a scanning Laser Doppler Vibrometer (LDV). This will then be analysed using Bayesian machine learning technology. The work will consider the combination of Bayesian identification of the physical model of wave propagation with a corrective machine learning component [1]. Two different technologies will be considered for this modelling. First a Bayesian hierarchical model will be fit constructed from known wave propagation models and Gaussian processes (GPs a flexible machine learning regression tool [2]). Secondly, a ‘likelihood-free’ approach will be adopted, this will look at how tools like Approximate Bayesian Computation (ABC) [3] could be incorporated into the guided wave modelling process when the exact models are not known.
The outputs of these models will inform strategies for damage detection in composite wind turbine blades. For example, this may include determining the probability of a defect/damage at any given location on the blade [4].
Importantly, the project will address the question of how to validate these models and how to determine a minimum viable sensor network which can be tied to the desired minimum level of uncertainty in the predictions, i.e. how many sensors to locate damage within 10cm vs 1cm. The damage detection methodology will follow a model-based SHM paradigm [5], however, the novelty in approach will be generated by using models which are not solely physical, instead which are augmented by machine learning components and which are inherently stochastic.
A successful outcome of this project would be to demonstrate the ability to detect, localise and quantify small defects in composite wind turbine blades using in-service ultrasonic inspection. This would be a powerful component of a digital twin based predictive maintenance system.
References & Further Reading
[1] Gardner, P., et al. “Learning model discrepancy: A Gaussian process and sampling-based approach.” Mechanical Systems and Signal Processing 152 (2021): 107381.
[2] Williams, Christopher K., and Carl Edward Rasmussen. Gaussian processes for machine learning. Vol. 2. No. 3. Cambridge, MA: MIT press, 2006.
[3] Abdessalem, Anis Ben, et al. “Recent Advances in Approximate Bayesian Computation Methodology Application in structural dynamics.” (2017).
[4] Fuentes, R., et al. “Autonomous ultrasonic inspection using Bayesian optimisation and robust outlier analysis.” Mechanical Systems and Signal Processing 145 (2020): 106897.
[5] Worden, Keith, et al. “The fundamental axioms of structural health monitoring.” Proceedings of the Royal Society A: Mathematical, Physical and Engineering Sciences 463.2082 (2007): 1639-1664.